The debate on crypto currencies rages on. The most popular debate hovers around the questions of broader acceptability of cryptocurrencies, whether at some point in time cryptocurrency usage can become so widespread that they start challenging the stature of fiat money? Would central banks bring cryptocurrencies under their regulatory ambit? Whether cryptocurrency is just another bubble, which will fizzle out as cost of funds creeps up, from level zero? With respect to the underlying technology, which enables cryptocurrency-namely Distributed Ledger Technology (DLT), there is more consensus on the brilliance of the dataset architecture and its potential. Blockchain, which has caught on popular imagination, is a specific dataset architecture within the broad class of architectures classified as DLT. The most popular used case of Blockchain being the cryptocurrency Bitcoin or rather the facilitation of transactions with Bitcoin.
There is a significant amount of anticipation on potential use of DLT other than cryptocurrency. These typically involve using DLT for facilitating high frequency transactions such as payment, manipulating transactions data, post trade settlement and the like. While DLT in its current avatar is close to a decade old however it continues to fall short of expectations in terms of basic performance parameters such as speed, scalability and operational efficiency. This has limited instances of widespread industrial scale implementations of DLT, despite generating promising results in ‘proof-of-concept’ stage in several cases. Given the current stage of development of the technology, it may be argued, whether such high-frequency fast response processes are the most optimal use of DLT. In this regard, conventional data base technologies on the lines of RDBMS handles way more volumes, more efficiently at higher speed. However, comparing the weakness of DLT with the strength of conventional database architecture may not be fair.
Among the strengths of DLT is its ability to enable peer-to-peer transactions without the need for a centralized monitoring/administrative entity. Of course, conventional data architectures do not support such functionality. So DLT may require used cases which leverage its strength. It has potential to be used for facilitating solutions to far wide ranging economic challenges which previously did not have the required technical infrastructure. One such use case is technologically enabling an International Currency Union where a global reserve currency may be mined by all member nations ie; ‘Mineable’ Global Reserve Currency-MGRC.It may be called a Worldcoin. Of course, it would require huge global political consensus ( or a major foreign currency crisis), to initiate the thinking and debate in that direction.
The argument for the desirability (or undesirability) of a new global reserve currency or a new global monetary order is outside the scope of the current piece. As such, the period from 1971 to 2008, which was characterized by the fiat currency form of US dollar as global reserve currency, had experienced high global growth with relatively lesser number of financial crisis/instability than most periods in history. Only the period from 1945 to 1970, had possibly experienced higher financial stability and more balanced growth than the period 1971 to 2008. However, the 2008 crisis ,and its aftermath, challenged quite a few economic assumptions including the existence and concept of ‘a’ global reserve currency issued by a sovereign i.e.; USA. Global policy response to 2008 crisis ranged from Unconventional Monetary Policy (UMP) to fiscal austerity. The jury is still out on the success of these measures, though strong expectations remain of a global economic recovery. The debate of global reserve currency revived because the trajectory of US economy and strength (or weakness) of USD has taken the world economy on a roller coaster ride. Particularly vulnerable being the emerging markets and developing markets .Such countries face strong challenge to improve the quality of lives of economically weaker sections and such efforts get sidetracked in the event of external financial shocks.
Arguably, regulators and fiscal policy makers have exhausted their box of economic tools with which to fight future financial crisis as well as address structural issues such as global fiscal and trade imbalance. We, given this background, create a case for usage of technology enabled economic tools such as a ‘mineable’ global reserve currency (MGRC), not issued by a sovereign or a group of sovereigns (example Special Drawing Rights-SDR). This MGRC may leverage DLT dataset architecture, while harnessing available enhanced computation power and big data capability to capture ‘live’ data on global trade and financing to facilitate algorithms, which will enable mining of the proposed Global Reserve Currency (GRC). Of course, some bitcoin enthusiasts have been, for some time, propounding that bitcoin itself may be a GRC but it is bit far-fetched unless it has wider public acceptance and explicit backing from governments.
Very Brief History of Global Reserve Currency and Foreign Currency Regime
Prior to World War I, it was the era of “commodity money”. The value of the money was driven by the value of gold, silver or copper contained in the coin. Exchange value of coins issued by different countries was driven by the inherent value of the coin i.e. quantity of the commodity in the coin and the ongoing price of the commodity. As such, the volatility of foreign currency exchange was lesser. In fact, because of the commodity nature of the coins the exchange rate to an extent was delinked from the underlying fundamentals of the economy that issued them.
When in mid-19th century paper notes started replacing commodity coins more widely, the paper coins retained the essence of commodity money. The paper notes were backed by promise of the issuing government/authority to replace it with gold/silver. The value of the paper note was driven by the amount of gold/silver that was promised by the issuing authority. This often introduced volatility or run on a currency, when the users of the note doubted the government’s ability to replace it with gold/silver and went ahead with demanding the commodity promised in the coin. In normal times, the exchange rates of such paper notes was driven by the amount of commodity the respective issuers/government promised with volatility getting introduced in the exchange rate in instances where people doubted the ability of the issuer to honor its commitment.
The First World War (WWI) ended commodity money when Great Britain, the issuer of sterling-the global reserve currency of the period, suddenly discontinued specie payment i.e. the bank notes ceased to be exchanged for gold coins. Post WWI financial instability aggravated and an effort was made by Britain in 1925 to return to gold standard, only to finally withdraw it in 1931. And thus Pound Sterling, which was gradually losing its stature as a global reserve currency to US Dollar, started to float.
The next pit stop in this story was the Bretton Woods Conference 1944. The Bretton Woods Conference debated on two proposals for a global monetary and foreign exchange regime. One was proposed by Harry Dexter White of USA and other was by John Maynard Keyes of United Kingdom. Keynes proposed an International Clearing Union (ICU), which would have issued a universal monetary unit of account called Bancor. ICU would be a multilateral body, keeping account/issuing Bancor, and thus no single country would have an overarching influence on its functioning. More details on ICU and related proposals are discussed later. The conference ultimately adopted White’s proposals, which among other things had a Stability Fund, which would have provided for much needed post war reconstruction of Europe. White’s proposal suggested that currencies have a fixed exchange rate against US Dollar which in turn would be convertible to gold. The proposal banked on member countries to issue currency to the extent of its gold ownership so as to maintain its exchange rate with respect to USD. For small deviations or imbalances, International Monetary Fund (IMF) was expected to step-in with support.
Between 1944 and 1970 there was a period of relatively high financial stability and growth particularly among the signatories of the agreement. USD was formalized as the global reserve currency. However, on August 15, 1971, US unilaterally discontinued the convertibility of USD to gold. With this, the USD became the first fiat money, not backed by gold, to become the global reserve currency. And it continues till date.
Post the Global Financial Crisis of 2008 when the balance sheet of US Federal Reserve expanded due to unconventional monetary policies including quantitative easing, it drove global monetary policy making to unchartered territory. Trade imbalances which was already on the rise pre-2008, surged. Currently, it appears that the health of emerging nations depends on US continuing its high fiscal deficit. The moment there is any plan/discussion in US on correcting its fiscal position or reducing the Fed’s balance sheet, there is often a capital flight of differing intensities in emerging markets. At some point in time, in not so distant future, central bankers and global regulators would need to find a way out of this dilemma. However, it appears that post-Keynesian / Monetarist /Neoclassical economics may not be having potent tools in its toolbox to handle this challenge.
Alternatives to current Global Reserve Currency- from Keynes to Stiglitz
Gessell to Keynes: The idea of a global reserve currency, which is issued by a supra-national entity as opposed to a sovereign in neither new nor recent. Back in 1920, Silvio Gessel, an economic thinker, proposed an institution called IVA-International Valuta Association, which was to issue a global monetary unit called Iva. The most prominent proposal on alternate monetary system was devised by Keynes and subsequently proposed at the Bretton Woods conference as UK’s official proposal. The basic tenet of Keynes’ proposal was the establishment of an International Clearing Union (ICU) based on an international bank money called Bancor meaning bank gold (in French). Bancor was proposed to be accepted as equivalent of gold for settlement of international balances. The union would not be linked to any one country but would be run as a multilateral agency.
Keynes realized that being the issuer of global reserve currency, as Pound Sterling was prior to 1940, was not an unmixed blessing. It almost always pushed the issuer to have unsustainable level of current account deficits with its ensuing deflationary overhand on the economy. Apparently, the seigniorage that the global reserve currency issuer charged to other countries came with a price.
Countries could hold Bancor as reserves as opposed to holding sterling, dollar or gold as reserves. The immediate benefit being, resources that remain trapped and thus passive as reserve could be released for funding real economic activity. The countries could, depending on their trade position, lend or borrow from the ICU. The union was expected to function as a supranational bank, where the credit of one country may be lent out to a debtor country. While similar financing transactions can be done using bilateral agreement, having a supranational quasi banking union can perform this function more seamlessly for pure economic considerations as opposed to possible political overhang which sometimes are inherent in bilateral financing transactions. The plan proposed a Governing Board to enforce fiscal and monetary discipline among the members.
The plan was intended to have a stabilizing impact on countries with payment or fiscal imbalance. In case of a surplus or deficit of even a quarter-year, the country would have to pay the ICU a charge of 1% per annum. Thus explicitly penalizing members for imbalance. If countries with deficit wants to extend their deficit by more than a quarter then the country needed to seek the Board’s permission. If the deficit exceeds a pre-determined threshold, the country may de-value its currency with respect to Bancor, to the extent of 5%- above which it would again have to seek the Board’s permission. The Board would be responsible for providing guidelines to countries to regain their trade balance.
Certain aspects of the proposal were difficult to implement then as it is now. However, the underlying construct of the plan as well as quite a few operational aspects of the plan continues to remain relevant if one considers a global reserve currency issued by a supra-sovereign institution. In Keynes’ original plan, founding members at initiation stage will set the value of their currency in terms of Bancor/Gold. Subsequent members would have to set the exchange rate in discussion with the board. Significant changes on exchange rate would have been allowed only with permission of the board. Likewise countries with surplus, which exceeds a pre-determined threshold for a quarter, would have to consider appreciating its domestic currency against Bancor. Alternately they could consider expanding its domestic credit or increasing money wages.
The idea may appear ‘unbelievable’ in this day and age of market driven foreign exchange rate. However, it must be remembered that market driven foreign exchange rate is a phenomenon, which came into being in 1970s after US Dollar was delinked from gold and became a free float. Even today absolute ‘value’ of a currency is not determined, one at best estimates the relative value of a currency against a basket of currencies or estimates likely future exchange rate of a currency with respect to another currency based on their respective inflation rates. And even in the last four decades no unambiguous framework exists which will tag a currency as ‘over-valued’ or ‘under-valued’ the way one would describe the value of equity of a company.
Keynes’ proposal included formula for calculating maximum allowed debit balance which was supposed to be the country’s quota. It will be based on the country’s export and import for last three years. Keynes also highlighted other factors to fine-tune the calculations. For 1940s this was computationally intensive but in 2020s with its ability to capture real time trade data and cross border financing data, one may not only implement more involved rules for quota calculation but clearly it is within the domains of technological possibility.
For quite a few years, it appeared that the global trade imbalance would cause a breakdown in international relations and trade; but nothing of that sort happened. However, the world needs options to move out of this current imbalance, considerations for an ICU-like framework may be relevant. In fact in the last couple of decades, particularly post 2008, several economists and thinkers have proposed frameworks which are based on ICU or frameworks which will achieve similar objectives.
Paul Davidson and International Monetary Clearing Unit: Paul Davidson improved on Keynes’ plan in an effort to make it more relevant to the economic and international political realities post 2000; since he did not consider the supranational central bank architecture suggested by Keynes to be either feasible or for that matter necessary.
His solution was for a “closed, double-entry bookkeeping clearing institution to keep the payments ‘score’ among the various trading nations plus some mutually agreed upon rules to create and reflux liquidity while maintaining the purchasing power of international currency”. On the lines of Gesell’s Iva and Keynes’ Bancor , Davidson proposed ‘International Money Clearing Unit’(IMCU) which will be the global reserve currency to be held only by the central banks of the member countries. ICMU can be transacted only between central banks and International Clearing Union. The private transactions will be cleared between the central banks accounts held with the clearing union. This is analogous to how money from one account-holder in a bank gets transferred to another account-holder in another bank through clearing facilities and involves adjustments against the reserves/deposits of the banks with the central bank.
The exchange rate between ICMU and local currency will be fixed and as per Davidson’s proposal will alter if efficiency wages change. ICMU value in local currency terms will also be driven by domestic inflation rate. This is to control for undue volatility of foreign currency and also reduce speculative attacks on currency. For excessive imbalances a country would have to take steps similar to Keynes’ plan.
Global Greenback Plan: Joseph Stiglitz and Bruce Greenwald proposed a Global Greenback system which was per se not a new global reserve currency but using the ‘Special Drawing Rights’ (SDR), as international reserve. However to the extent SDR is itself a basket of six currencies (latest addition being Chinese renminbi) a lot of the shortcomings identified by Keynes with respect to a global reserve currency being issued by a single sovereign would hold.
Thus, if the world ever goes more a mineable global reserve currency it is likely to have features closer to Keynes’ Bancor or Davidson’s ICMU.
Basics of DLT and Cryptocurrency[1]
DLT is a class of database architecture. A specific type (and of course the most talked about) of DLT is Blockchain. Blockchain caught onto popular imagination because it enables Bitcoin, the most popular cryptocurrency. DLT allows for accessing and updating database from multiple nodes (i.e.; Computers/CPU associated with a user/users) which may be in geographically different locations . The integrity/accuracy of the database is ensured through validation procedures which require consensus of all or some select nodes. As such, the concept of distributed access of a database or simultaneous access across physically different locations is neither new nor unique to DLT. In conventional databases, the storage of data could have been spread across multiple computers and accessed across different nodes. However there was one version/one view/one instance of the dataset at any point in time. The integrity/accuracy of the data depended on centralized data administration capability. Requests for addition to the database or updating existing records would be vetted/validated by data administration before it got reflected in the database. The centralized data administration capability may be called a ‘trusted authority’.
So a payment facility whose IT infrastructure consists of conventional database (which, as of today, is almost all) is likely to operate as follows. When a payment is made the payer’s account is debited and the payee’s account is credited and the system is updated to reflect the updated account status. The trusted authority is responsible for ensuring that the system correctly and promptly captures the transactions and reflects the latest account position. Other authorized, systems can access the central database to find the state of the payer and the payee
The ‘problem’ of course is that the trusted authority has tracked the transaction i.e. the transaction is not anonymous. In DLT there is no trusted authority i.e. no centralized facility to ensure data integrity. In that sense a ‘Distributed’ Ledger is sometimes called a ‘mutually distributed ledger’ to underline the fact that the ownership of data integrity is on the entire set of participating nodes and not just on some centralized data administrator.
In DLT, the users (node) of the distributed system each have a copy (instance) of the entire database. Once a new transaction event occurs, it has to be updated at each of the instances, which may be accessed by one or more nodes. A transaction is successfully ‘updated’ when each of the nodes has given their consent to the transaction. A transaction event finally gets reflected in the database or rather all instance of the database when all nodes with validation authority have accepted the transaction as valid. As such this process is time-consuming and even when a bona fide transaction event happens, there is only a probabilistic finality of settlement. In many situations, the final settlement can actually take several hours.
Within DLT, the specific workflow, which enables the consensus/validation, differs leading to different type of DLT. As such a validation framework based on simple majority (with each user/node having one vote) would expose the system to attackers who would simply proliferate nodes to game the system. To take care of such possibilities, complex validation protocols were developed. One framework of validation is ‘Proof of Work’(POW) where the ‘proof’ consists of solving mathematical problems requiring significant computation power, thereby increasing the hurdle of one or a small number of attackers to game the system. Another competing validation framework is a “Proof of Stake”(POS) based consensus . The rights of validations of the nodes depend on their stake in the system. The measurement of stake also has several alternatives in the sense the stake can be measured by the number of token/cryptocurrency the nodes own or number of units of cryptocurrency the node is willing to bet on that transaction.
While thus far we would have got an idea of the distributed nature and technological aspects, one question still remains unexplored. Why is it called a ‘ledger’ as opposed to a database? Ledger usually refers specifically to a functionally of a database where one keeps track of which entity owns how much on a product/value/unit and subsequently how those units are getting spent or further units accumulated. The concept of Ledger is very critical for a non-physical/digital currency, operated in absence of a trusted authority, to prevent double-spending of the same unit.
Efficiency and Choice of Access and Validation: A DLT can be further characterized on two dimensions: i) Accessibility and ii) Validation Right. In terms of accessibility the options are broadly two namely Public, where any user is allowed to read/view the ledger and Private, where access is limited to users with explicit access rights. Validation rights refer to who can validate or make changes in ledger. Here the two options are, permissioned where only a subset of all users who are specifically identified can validate/modify the ledger. The other option being Permission-less where all users are allowed to add/alter/validate the ledger
The way popular cryptocurrency transactions are facilitated by Blockchain typically follows public, permission-fewer frameworks. Needless to say this process takes a lot of time and computation power. From a user perspective when bank money is transferred, it takes seconds or few minutes to confirm the transaction to the parties. In DLT the transaction confirmation sometimes takes hours. Financial services are used to electronic payment networks which process upwards of 25,000 transactions per second. In comparison large block chain network processes less than 10 transactions per second.
However, for the purpose of a mineable Global Reserve Currency it may be envisioned that it will be a Private and Permissioned protocol, so speed of the transactions may not be an issue. As such an idea such as MGRC may take at least a couple of decades to implement unless of course there is a major crisis pushing global consensus on such a move. While the technology around DLT and Blockchain is close to a decade old and certain commentators still consider the scalability and power usage as a bit underwhelming, it may be hoped that these issues will be resolved with time.
Economics of Cryptocurrency: Currency is a subset of a broader economic concept of money. Currency is the ‘token’ which facilitates movement of money, shifting its purchasing power from the current holder to its future holder. Currency facilitates the exchange feature of money i.e. payment. Currency, driven by technology has evolved into several forms; from good old cash/coins, to electronic money and more recently cryptocurrency. Currencies are typified by key features namely physical existence, issuer, ultimate liability, universal accessibility and peer-to-peer exchangeability. Cash ticks most boxes i.e. exists physically, issued by central banks with ultimate liability being that of the government, universally accessible within the jurisdiction and can be anonymously ,at zero cost, used in peer-to-peer exchange. Electronic money represents electronic payments facilitated by banks, payment networks differ from cash as they do not have physical existence and finally, peer-to-peer exchange are tractable by authorities and hence anonymous like cash.
A Cryptocurrency addresses the need to enable peer-to-peer anonymous transaction on the lines of cash. However cryptocurrency provides this anonymity in online/electronic transactions which credit card/debit card/internet banking based payments cannot. This explains the ‘crypto’ aspect of the cryptocurrency.
Suggested Framework of a Mineable Global Reserve Currency (MGRC)
The first version of Basel norms (Basel I) had, in comparison to the current banking regulations, relatively simpler framework for capital requirement. Still it took 14 years of negotiation (started in 1974, as Basel Committee on Banking Supervision) by central banks of the group of 10 countries (7 European, USA, Canada and Japan). These countries had largely similar econo-political orientation. If typical WTO negotiations are anything to go by, a coordinated, globally synchronized effort to create a new monetary framework would easily take a couple of decades. Previous instances of synchronized effort to shift to a new monetary regime took earth-shattering events like a couple of World Wars. So technological feasibility of a MGRC is the least of the roadblocks. However, the frequency and intensity of the debate around the MGRC can increase since it is an issue of global political intent and not of technical ability.
The proposed framework is dependent on the current state of technological sophistication of the DLT. It is not expecting any significant improvement on the same. Since Keynes had designed the first detailed framework, the proposed framework, in his memory, may be called International Currency Union-ICU and the MGRC may be named as WorldCoin
Governance Structure of ICU: The ICU may consider a four-tiered administrative structure. The overall enablement and infrastructure support may be under the Governing Board. The Board would have representatives from all members; however a sub-group selected by rotation may make the core decisions on an ongoing basis. The stewardship of the core decision-making group may be rotated on a periodic basis among member countries. The Board would interact directly with the respective Central Banks of the member nations.
The central banks would be responsible for maintaining the accounting and cadence framework, which will justify the conversion rate of local currency with respect to MGRC. Most importantly, the central banks are the entities who will be responsible for actually ‘mining’ the MGRC based on the trade and macro parameters. Central banks are best placed to do so since in most countries they are directly responsible for determining their domestic money supply/credit supply, which ultimately influences inflation and growth.
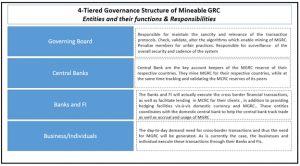
Banks and Financial Institutions regulated by their respective central banks will facilitate the transactions in MGRC. Specifically, these regulated financial institutions will be responsible for executing cross border financial transactions where the underlying is MGRC. Foreign currency transactions being facilitated by domestic banks is also the case today, under the proposed scheme of things the foreign currency will be replaced by MGRC.
Operational aspects of accruing and accounting MGRC: The central banks would be responsible for keeping track of the MGRC account with respect to its country. The MGRC reserves of a country will keep on fluctuating constantly, for reasons pertaining to business and economy, which will largely be same set of reasons which cause fluctuation in value of current reserve holdings. Currently, typical reserves of most countries constitute some combination of USD, SDR or currencies in SDR and Gold. An incremental source of accruing MGRC will be ‘mining’ the MGRC based on positive trade/cross country financial trade data.
At the lowest level (Level 1), demand (outflow) and supply (inflow) for MGRC will be attributable to trade of Goods/services between businesses/individuals domiciled in different countries. A net positive export (by value) will cause the country to earn(net) MGRC and increase its MGRC holding while the opposite impact will happen if the country is a net importer. The trade (payment aspects, specifically) will be facilitated by banks and financial institutions, as is the case today. Under a proposed MGRC framework, the business will earn or spend in terms of MGRC (as opposed to, say, USD/EURO/JPY today) and they may be allowed to hold MGRC accounts with the bank. For calculation of profits or financial reporting purposes, the conversion rate of MGRC with respect to the local currency needs to be used. For that matter if a business/individual wants to convert its/his MGRC holding into local currency, and vice-versa, it can be execute based on the conversion rate.
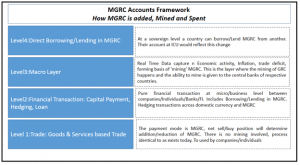
The Level 2, consists of financial institutions which will facilitate international trade related payments. This intermediation function will not incrementally alter the MGRC reserve of the country over and above what is contributed by the underlying business and trade. However, to the extent these financial entities start providing MGRC related hedging tools (against change in conversion rate of local currency vis-à-vis) and loans denominated in MGRC, they will affect the MGRC reserve of the country. For the MGRC loans extended to businesses/individual by their respective domestic banks, the domestic bank may disburse the MGRC loan from various sources such as its own MGRC account, it may borrow from the central bank, or it may borrow MGRC from another domestic and international bank for onward lending. This is also the layer, which will absorb the capital inflows/outflows. Thus if FDI flows into a country, denominated in MGRC, this is the layer which will facilitate the monetary elements of the transactions
Level 3 is the layer where only the central banks of respective countries operate. Central banks constitute the only entities which are allowed to mine the MGRC and add to the country’s MGRC. The mining algorithms may be determined by macro factors’ such as inflation and fiscal deficit. Recall separate capital account deficit or trade deficit need not be considered in the mining algorithm as their impact on MGRC reserve will be deterministically captured in Level 1/Level 2 .
Level 4 is the topmost layer, which can add/reduce the MGRC reserves. This is bilateral borrowing between nations. For example, nations whose demand of MGRC is rising much faster than their ability to earn the same and who have weak economic parameters which prevent them from mining new MGRC, the MGRC will appreciate sharply against their domestic currencies. In such cases the country in crisis may borrow MGRC from another country with comfortable MGRC reserves. Such borrowings would increase the MGRC reserve of the borrower and reduce MGRC reserve of the lender.
Operational Details of Cross-Border MGRC Transactions: If a business in a country wants to make a payment to a foreign company/individual through MGRC, the set of transactions will be analogous to the transfer of money from one bank account to another bank across the border. In the current framework, the DLT will enable live tracking of the MGRC account at not only the business or bank level but it will get updated at the Central Bank level, so that ultimately the Governing Body of the ICU knows the MGRC holdings at country level. Additionally the countries are aware of each other’s MGRC level that will build higher level of trust and would address issues of currency manipulation.
Conclusion: The option to have a Global Reserve Currency, which is not issued by any one sovereign, needs to be actively discussed. In the scheme of things, the entire world paying seigniorage to a single country is possibly the least of the issues. As Keynes has identified the problem with a single sovereign issuer of reserve currency, which used to be the case with UK and Pound Sterling prior to 1930, the system can operate ‘well’ only when the issuer country runs a large deficit. This imbalance attributable to continuously running deficits, even in absence of a major economic shock, will push the issuer towards a deflationary economic environment. In the event of a major crisis such as in 2008, US had to resort to unconventional monetary policy in a bid to prevent a repeat of the 1930s recession. Not just US, issuers of major currencies such as Pound, Yen, Euro have adopted some version of unconventional monetary policy. These countries will gradually try to move towards normal monetary environment (i.e., non-zero interest rate, no excess liquidity via quantitative easing) This ‘normalization’ of monetary policy may reduce funding liquidity, and also limit credit/money supply. The steps and the outcome are largely outside the domain of usual hypothesis/deductive reasons applicable to prevalent macro thinking. It may be fair to assume that each of these countries will try to safeguard their own national economic interest before anything else.
If the unwinding of the existing near zero interest regime and normalization of a liquidity deluge environment is relatively painless for other countries, then possibly there will be not much trigger for discussion of a country neutral GRC. However, if there are widespread market disruptions or economic shocks, that may provide an opportunity to discuss GRC, which is independent of the fortunes of an issuer country. In fact, a country-neutral GRC may prevent foreign currency contagion in emerging nations. It would of course mean a shift towards a new monetary regime globally and may potentially challenge or disrupt the functioning of FX currency markets particularly its most important constituency- FX traders.
The DLT comes closest to providing the ideal technological infrastructure for MGRC. To enable transparent, real-time tracking of reserves as well as ‘mine’ the reserve currency without day-to-day centralized intervention by any authority, a Blockchain like data architecture may be useful. Apart from building trust in the system it will reduce dependency on the economic fortunes of any one country. To the extent that the Central Banks themselves will be the direct users of this peer-to-peer system, the information is unlikely to be used by manipulative elements in currency markets.
As far as using the concept and framework of MGRC is concerned, the framework can be tweaked to create a two-tiered monetary structure say for instance in Eurozone. Where the Euro can become a currency of exchange in trade in Eurozone (as of course is the case currently) but each country have their own currency mapped to a ‘Mineable’ Euro. In a relatively smaller scale, the same framework can be used by multilateral trade bodies to keep track of accounts/as well as payments based on mineable currency as opposed to using global reserve currency or the currency of the largest trading member in that trade bloc. The MGRC may possibly, if and when implemented, turn out to be the most important use case of DLT.
Reference
Morten Bech & Rodney Garratt; Central Bank Cryptocurrency; BIS Quarterly Review, September 2017
David Andolfatto, Bitcoin and Beyond: The possibilities and pitfalls of virtual currencies, Federal Reserve Bank of St.Louis, March 2014
Joseph Bonneau, How long does it take for Bitcoin transaction to be confirmed, Coin Centre, November 2015
Gareth W. Peters et al; Trends in Cryptocurrencies and Blockchain technologies:a monetary theory and regulatory perspective, The Journal of Financial Perspectives Winter 2015
M.Raskin and D.Yermack; Digital currencies, decentralized Ledgers and the future of central banking; NBER Working Paper, May 2016
Josh Ryan-Collins,Tony Greenham,Richard Werner,Andrew Jackson; Where Does Money Come From?
John Keynes; A Treatise on Money
[1] Readers, aware of the basics of DLT and Cryptocurrencies, may skip this section without loss of continuity.