Efficiency of any audit market is broadly measured by quality of audit. Audit quality, in turn, depends on two factors- (a) ability to identify misreporting and errors in financial statements; and (b) willingness to report such errors and/or misstatements. While the first factor relies on the skill and competence of auditors, the second one surely depends on independence of auditors. It is generally believed that large audit firms can ensure better audit quality as they employ more competent resources who have requisite auditing skill as well as knowledge of select sectors or industries. Smaller audit firms could not afford large number of efficient auditors for cost reasons. As a result, audit market internationally is dominated by the oligopoly of the so-called Big 4 audit firms (Deloitte, EY, PwC and KPMG). The evolution of audit market over the past three decades showed further concentration of the industry- from Big 8 auditors to Big 4 auditors (see figure in next page). Is such a level of concentration good for the audit market? Will this feature make the audit market less efficient? Answer to these questions lies in quality of audit rendered by Big 4 and other auditors. Whether Big 4 auditors provide higher audit quality is an empirical questions and evidences are mixed. Simunic and Stein (1987) suggest that having spent heavily on building their brand names, the Big 4 auditors have an incentive to protect their reputations by providing more credible financial reports. Another study (Becker et al, 1998) shows that Big 4 auditors were able to restrain audit clients from earning manipulations. Jaggi et al (2014) confirm firms audited by industry specialists reflect a better earnings quality compared to audits by non-specialists. There are counter evidences too. Lawrence et al (2011) find that the effects of Big 4 auditors on audit quality are insignificantly different from those of non-Big 4 auditors.
The second issue concerning efficiency of audit market deals with auditor independence. Auditor tenure defines the relationship between auditor and auditee. Longer auditor tenure has mixed implications. On one hand, it allows audit firms to gain deeper knowledge about the client and thus helps the auditor ensure better audit quality. Skeptics, on the other hand, point out that longer auditor tenure creates problem with entrenchment of auditor, compromising the quality of audit. Auditor independence refers to the ability of auditors to be free from persuasion, influence or bias. One popular measure of auditor independence is the size of audit fees. Proportion of audit fees to non-audit fees is also used as a proxy for auditor independence (Frankel et al. 2002). Accounting firms argue that providing consulting services to audit clients produces knowledge spillovers that increase audit efficiency and hence questioning auditors’ independence in such situation is not valid. Others observe that non-audit fees billed to audit clients are positively associated with proxies for earnings management.
Fees capture both demand and supply factors associated with audits. However, one may question that oligopolistic premium charged by Big4 auditors may not translate to better audit quality. It is believed that auditor independence can be ensured through (a) periodic rotation of auditors and (b) regulating the type of consulting services that an auditor can render for the audit client.
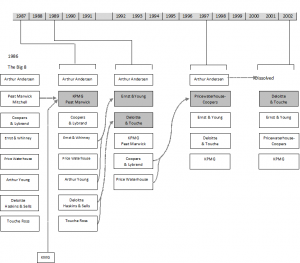
Source: Patrick Velte and Markus Stiglbauer, Audit Market Concentration & Its Influence on Audit Quality. International Business Research. Vol 5, No. 11, 2012.
Audit Market in India
Audit market in India is characterized by three features- dominance of Big 4 auditor, increasing share of non-audit fee and advocacy for joint audit. Dominance of Big 4 audit firms in Indian audit market is not new. They began to grow in the Indian market since 1991 (Desai et al., 2012). However, the Big 4 multinational audit firms are not permitted to audit accounts under their own name and therefore they formed networks with local Indian audit firms to conduct audit in India (Layak & Mehra, 2009).Table 1 shows the number of companies audited by Big 4 auditors and top non-Big 4 auditors for past three financial years. The top ten audit firms accounted for audit of 36% of listed companies in NSE during 2015-16. Within the Nifty 500 subset, the dominance of Big 4 Auditors was much higher. In financial year 2015-16, they handled a total of 234 audits (46%). The overall audit market in India is crowded with a large number of small single-location audit firms. For example, 830 audit firms audited 1519 NSE-listed companies in 2015-16 with an average volume of less than 2 auditee per auditor[1].
TABLE 1
Number of Company Audits
Auditor Name or Group[2] |
# of Companies
audited in 2015-16 |
# of Companies audited in 2014-15 |
# of Companies
audited in 2013-14 |
Big N Auditor
(4 firms) |
403 |
380 |
371 |
Non-Big N Auditor
(6 firms) |
157 |
149 |
137 |
Note: If a client has joint audit, credit has been given to each auditor
The total audit fee paid out by companies was a significant Rs. 19,000 million[3] during 2015-16. This was an increase of 9 per cent from the Rs. 17,460 million paid out in the previous financial year 2014-15. The average audit fee paid by 1400 NSE-listed companies was Rs. 13.7 million. Table 2 shows the breakup of audit fees and total fees for last three financial years. The average audit fee earned by Big 4 auditors was Rs. 1,197 million- which was almost 90 times of average audit fees of the industry during 2015-16. There is a huge inequality in audit fee paid by large and small cap companies. About a quarter of the fees earned by Big 4 auditors came from non-audit services. Other services for Non-Big 4 auditors constitute 10% of total fees. Therefore, non- big 4 auditors are more dependent on audit fees. Big 4 auditors in terms of business volume and fees earned dominate Indian audit market. It may be interesting to examine whether audit quality in India has improved in such a dominant audit market structure.
TABLE 2
Breakup of audit fees
Auditor Name or Group |
2015-16 |
2014-15 |
2013-14 |
Total Fees |
Non- Audit Fees (%) |
Total Fees |
Non- Audit Fees (%) |
Total Fees |
Non- Audit Fees (%) |
Big 4 Auditor |
6614.8 |
28% |
5984.2 |
25% |
5404.1 |
27% |
Non-Big 4 Auditor |
2123.7 |
11% |
1769 |
17% |
1728.5 |
8% |
(6 firms) |
|
|
|
|
|
|
Industry Average |
13.7 |
|
12.1 |
|
|
|
Note: The figures above are in INR Million. |
|
|
|
|
Joint audit is voluntary in India. There are only 91 companies out of Nifty 500 subset practicing joint audit. Auditors in India (other than Big 4) were advocating for mandatory joint audit for large companies. However, the Government of India rejected the plea by auditors for joint audits in large companies stating that it is not a viable option for promoting domestic audit firms. Even if joint audit is mandated, it cannot be guaranteed that one small firm and one big 4 firm will handle the audit. Table 3 lists instances of voluntary joint audits of audit clients in Nifty 500 subset. About 10% of companies went for joint audit every year- these include banks and PSUs.
TABLE 3
Longitudinal distribution of firm-year observations
|
Audit Firm type |
Joint Audit type |
Year – Fiscal |
Big 4 |
Others |
Total |
Joint Audit |
# of firm-years in this year/ total # of firm-years |
2007 |
170 |
239 |
409 |
63 |
9.09% |
2008 |
176 |
235 |
411 |
62 |
8.95% |
2009 |
177 |
230 |
407 |
63 |
9.09% |
2010 |
182 |
226 |
408 |
74 |
10.68% |
2011 |
180 |
228 |
408 |
71 |
10.25% |
2012 |
183 |
226 |
409 |
74 |
10.68% |
2013 |
183 |
224 |
407 |
72 |
10.39% |
2014 |
180 |
224 |
404 |
71 |
10.25% |
2015 |
186 |
223 |
409 |
70 |
10.10% |
2016 |
191 |
205 |
396 |
73 |
10.53% |
Total |
1808 |
2260 |
4068 |
693 |
100% |
|
|
|
|
|
|
Compiled from Ace Equity Database.
Corporate Law and Audit Market in India
The new Indian corporate law (Companies Act 2013) seeks to address the audit quality issue from two angles- auditor rotations and prohibition in certain non-audit services to audit clients. Companies Act 2013 made rotation of auditors mandatory for listed and many unlisted companies effective from April 2017 in situations where an auditor has served in that capacity with a particular auditee for ten or more years consecutively. There is also a provision of a cooling period of five years for the audit firm with respect to the same audit client. One more important provision in this respect is about rotation of audit partner. The law states that no audit firm having a common partner(s) to the other audit firm, whose tenure has expired in a company immediately preceding the financial year, shall be appointed as auditor of the same company for a period of five years. It implies that if a signing partner of an outgoing audit firm (due to rotation) joins another audit firm, the latter audit firm will also be ineligible to be appointed as auditor of the same audit client in the immediate subsequent year. Consider an example, if Mr. X, signing partner of audit firm ABC (which has just completed ten consecutive years of audit of a client MNO) joins a rival audit firm PQR, the latter audit firm (PQR) will not be allowed to conduct audit of MNO at least for one year. However, if MNO approaches PQR after a gap of one year since ABC audited the company, PQR would have no restriction in accepting the audit client. Therefore Indian corporate law does not specifically require audit partner rotation.
Table 4: Auditor Rotation
Year |
Auditor Rotation |
Audit Firm Rotation |
2008 |
126 |
83 |
2009 |
106 |
58 |
2010 |
133 |
87 |
2011 |
140 |
81 |
2012 |
135 |
80 |
2013 |
127 |
59 |
2014 |
165 |
87 |
2015 |
144 |
75 |
2016 |
131 |
75 |
Average |
134 |
76 |
Auditor rotation was not mandatory under the earlier corporate law and yet it was in vogue in India (Table 4). Data on Nifty 500 over the past nine years show that annually 134 audit clients on average had different audit partners signing their financial statements (partner rotation). About 15% of the Nifty 500 companies had changed their auditor every year.
Auditor rotation is mandatory from the current financial year. Recent data on Indian audit market show that auditor rotation did not adversely affect volume of business of Big 4 multinational audit firms. For example, the top two of the Big 4 auditors in India (Deloitte and EY) have bagged enough new auditees from other two Big 4 and smaller auditors to compensate for the number of clients they lost due to mandatory audit rotation[4]. It has also been observed that large Indian corporate chose to just swap the auditors or replace an Indian auditor with one of the multinational audit firms. Therefore, the audit swap among Big4 firms also denied opportunities to local and smaller audit firms any additional business. There is no empirical evidence to suggest that auditor rotation leads to greater independence of auditors. In fact, there is greater possibility of bad audit in first year under any new auditor due to learning curve effect.
To ensure independence of auditors, another restrictive provision in the Companies Act 2013 relates to rendering of non-audit services. Services out of the ambit of a statutory auditor include internal audit, book keeping, investment advisory services, investment banking services, and management services. The restriction is much wider- it includes not only the audit client but also its holding or subsidiary companies. The term ‘management service’ is little vague and is nowhere defined in the Act. Therefore, it is not clear which non-audit services, other than taxation services, may be assigned to an auditor. Will this restriction improve auditors’ independence? Answer is not straightforward. One obvious argument in favour of such restriction is to ensure that auditors are not dependent on corporate largesse and hence can be forthright in expressing their opinion. The ‘resource-diversion’ view suggests that expanding consulting services could undermine audit quality. There is equally strong argument against such restrictive provisions. Providing consulting services may improve audit quality- consulting staff often provide valuable insights to the audit staff because they act as ‘domain specialists’ on audit engagements.
If one puts the above two restrictive provisions – auditor rotation and prohibition of non-audit services in perspective, a natural question would be whether these provisions would have negative effect on the overall income of Big 4 audit firms. Recent data on impact of mandatory auditor rotation in India showed that there was no reduction in audit clients of Big 4 firms due to audit swap. It is quite possible, and also perfectly legal under the new corporate law, that an audit firm retains non-audit services of a client while relinquishing audit services. Thus, Big4 audit firms would only swap their audit clients for audit services and retain non-audit services of the old auditees. Therefore, the restrictive provisions of the Companies Act 2013 may prove to be windfall for Big 4 auditors in India.
The Companies Act did not make joint audit mandatory. Rather the law left it to the shareholders of a company to decide about appointment of joint auditors. In case any company decides to have joint audit, the law states that the company shall follow the rotation of auditors in such a manner that all of the joint auditors do not complete their term in the same year.
References
Becker, C.L., DeFond, M.L., Jiambalvo, J. and Subramanyam, K.R. (1998), “The effect of audit quality on earnings management”, Contemporary Accounting Research, Vol. 15 No. 1, pp. 1-24.
Desai, R., Desai, V., Singhvi, M. & Munsif, V. (2012), ‘Audit fees, non-audit fees, and auditor quality: an analysis from the Indian perspective’, Journal of Accounting, Ethics & Public Policy, Vol. 13, No. 2, pp. 153–65.
Frankel, R. M., M. F., Johnson and K.K., Nelson, (2002). “The relation between auditors’ fees for nonaudit services and earnings management”, The Accounting Review, Vol. 35, No. 1, pp. 71–105
Jaggi, B., Mitra, S. & Hossain, M., (2014). Earnings Quality, Internal control weaknesses and industry specialist audits. Review of Quantitative Financial Accounting, pp. 5-32.
Lawrence, Alastair and Minutti-Meza, Miguel and Zhang, Ping, Can Big 4 Versus Non-Big 4 Differences in Audit-Quality Proxies be attributed to Client Characteristics? (June 16, 2010). Accounting Review, Vol. 86, No. 1, pp. 259-288.
Layak, S. & Mehra, P. (2009), ‘Inside the secret world of auditing’, Business Today, 22 February, pp. 54–60.
Simunic, D. A., and M. T. Stein. (1987). Product differentiation in auditing: Auditor choice in the market for unseasoned new issues. Canadian Certified General Accountants’ Research Foundation, Vancouver, B. C.
[1] Prime Database, September 2016
[2] Big N Audit Groups as per Prime Database: 1. Deloitte Group (Deloitte Haskins & Sells, Deloitte Haskins & sells LLP, A F Ferguson & Co, C C Chokshi & Co, Fraser & Ross, S B Billimoria & Co) 2. EY Group (S R B C & CO LLP, S R Batliboi & Associates LLP, S R Batliboi & CO LLP, S V Ghatalia & Associates LLP) 3. Price Waterhouse Group (Price Waterhouse,Price Waterhouse & Co, Price Waterhouse & Co LLP, Bangalore, Dalal & Shah, Lovelock & Lewes) 4. KPMG Group (B S R & Associates LLP, B S R & Co LLP,B S R & Company, B S R and Associates, B S R and co, B S R and company)
[3] Data based on 1,389 companies for which audit fee/total fee data was available. Source: Prime Database
[4] The Economic Times, June 14, 2017